Google Gemini: Multimodal AI Model Deep Dive
Google Gemini: Multimodal AI breakthrough. Learn how Google’s new AI model understands text, images, and more, impacting search, conversation, and beyond.
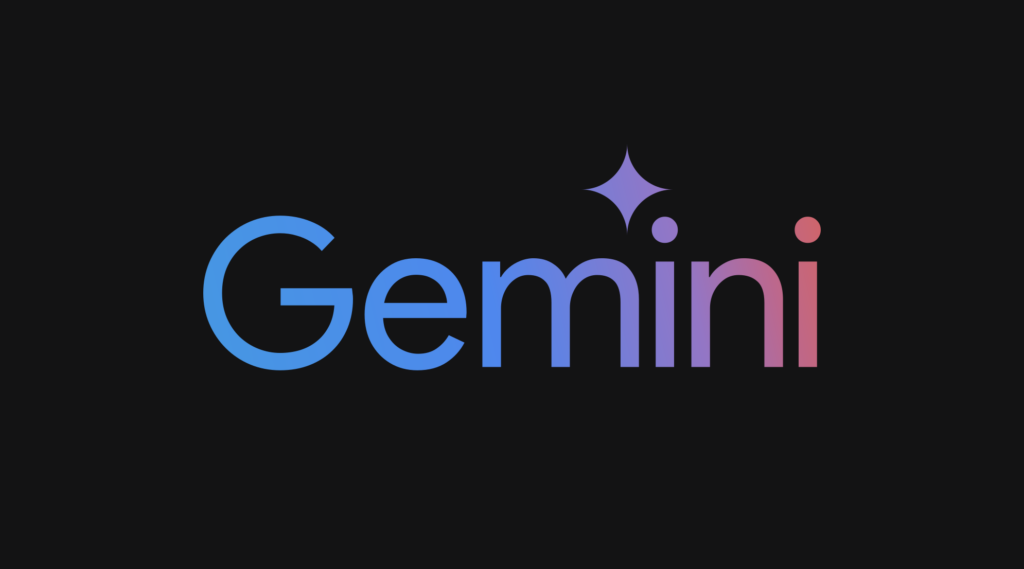
Google Gemini: A Deep Dive into Google’s Multimodal AI Model
Google Gemini represents a significant leap forward in artificial intelligence, marking Google’s ambitious endeavor to create a truly multimodal model.1 Unlike previous AI models primarily focused on text or images, Gemini is designed to understand and generate content across various modalities, including text, code, images, audio, and video.2 This article provides a comprehensive overview of Google Gemini, exploring its architecture, capabilities, potential applications, and the implications it holds for the future of AI.
The Need for Multimodal AI
Traditional AI models often specialize in a single modality. For instance, large language models (LLMs) like BERT and LaMDA excel at processing and generating text, while computer vision models like ResNet are adept at analyzing images. However, the real world is inherently multimodal. We perceive and interact with the world through a combination of senses, processing information from various sources simultaneously. Multimodal AI aims to replicate this human-like ability, creating models that can understand and reason across different modalities.3
Gemini is Google’s response to this need, aiming to create a more general-purpose AI that can seamlessly integrate and process information from diverse sources.4 This approach opens up a world of possibilities, enabling AI to perform more complex and nuanced tasks.5
Gemini’s Architecture and Key Features:
While Google has released some information about Gemini, full technical details are still emerging. However, based on available information, we can highlight some key architectural aspects and features:
- Multimodal Training: Gemini is trained on a massive dataset encompassing various modalities, including text, code, images, audio, and video.6 This diverse training data allows the model to learn relationships and connections between different modalities.7
- Transformer-Based Architecture: Like many recent successful LLMs, Gemini likely utilizes a transformer-based architecture, which is highly effective at processing sequential data and capturing long-range dependencies.8 This architecture allows the model to understand the context and relationships between different elements within and across modalities.9
- Emphasis on Reasoning and Understanding: Gemini is designed not just to generate content but also to understand and reason about the information it processes.10 This involves capabilities like common sense reasoning, logical inference, and understanding of physical laws.
- Different Sizes and Capabilities: Google has indicated that Gemini will come in different sizes, optimized for various tasks and platforms.11 This includes smaller, more efficient versions for on-device applications and larger, more powerful versions for complex research and development.12
- Integration with Google Products and Services: Gemini is expected to be deeply integrated with Google’s existing products and services, such as Search, Assistant, and Workspace, enhancing their capabilities and providing users with more intelligent and seamless experiences.13
Capabilities and Potential Applications:
Gemini’s multimodal nature opens up a wide range of potential applications across various domains:14
- Enhanced Search and Information Retrieval: Gemini could revolutionize search by allowing users to search using a combination of text, images, and voice. For example, a user could take a picture of a landmark and ask Gemini to provide information about its history and surrounding area.
- Improved Conversational AI: Gemini could power more natural and engaging conversational AI experiences, allowing users to interact with virtual assistants in a more human-like way.15 These assistants could understand and respond to complex queries involving multiple modalities.
- Advanced Content Creation: Gemini could assist in creating various types of content, such as generating descriptions for images, creating videos from text prompts, or even composing music based on user preferences.16
- Code Generation and Debugging: Gemini’s ability to understand code could lead to more advanced code generation and debugging tools, helping developers write code more efficiently and effectively.17
- Education and Learning: Gemini could create more engaging and interactive learning experiences, allowing students to explore complex concepts through a combination of text, images, and simulations.18
- Accessibility: Gemini could improve accessibility for people with disabilities by providing alternative ways to interact with technology.19 For example, it could generate audio descriptions of images for visually impaired users.
- Scientific Research: Gemini could assist in scientific research by analyzing complex datasets across multiple modalities, such as analyzing medical images alongside patient records.20
Comparison with Other Multimodal Models:
While Gemini is a significant advancement, it’s not the only multimodal AI model in development.21 Other notable examples include:
- OpenAI’s GPT-4: While primarily known for its text generation capabilities, GPT-4 also demonstrates some multimodal abilities, such as generating image descriptions.22 However, it’s not explicitly designed as a fully multimodal model like Gemini.
- Other Research Efforts: Various research labs and companies are actively exploring multimodal AI, developing models that can process and integrate information from different modalities.23
Gemini distinguishes itself through its explicit focus on multimodality from the ground up, its emphasis on reasoning and understanding, and its planned integration with Google’s vast ecosystem of products and services.24
Challenges and Ethical Considerations:
Developing and deploying multimodal AI models like Gemini presents several challenges and ethical considerations:
- Data Requirements: Training multimodal models requires massive amounts of diverse data, which can raise concerns about data privacy and bias.25
- Computational Resources: Training and running large multimodal models requires significant computational resources, which can limit accessibility and raise environmental concerns.26
- Bias and Fairness: Multimodal models can inherit and amplify biases present in the training data, leading to unfair or discriminatory outcomes.27
- Misinformation and Manipulation: The ability to generate realistic content across multiple modalities could be misused to create misinformation or manipulate public opinion.
- Job Displacement: The automation potential of multimodal AI could lead to job displacement in certain industries.
Addressing these challenges and ethical considerations is crucial for ensuring the responsible development and deployment of multimodal AI.
The Future of Multimodal AI with Gemini:
Gemini represents a pivotal moment in the evolution of AI, signaling a shift towards more general-purpose and human-like intelligence.28 Its multimodal capabilities have the potential to transform various industries and aspects of our lives. While the full extent of Gemini’s capabilities and impact remains to be seen, it’s clear that it will play a significant role in shaping the future of AI.
The development of Gemini also underscores the ongoing race in the field of AI, with major tech companies investing heavily in research and development.29 The advancements made with Gemini will likely inspire further innovation and accelerate the development of even more sophisticated multimodal AI models in the future.
In conclusion, Google Gemini is more than just another AI model; it’s a testament to the growing ambition to create AI that can truly understand and interact with the world in a human-like way. By bridging the gap between different modalities, Gemini opens up a world of possibilities, paving the way for a future where AI plays an even more integral role in our lives.